Return to Discussion Forum
Title: Topics - Face-to-Face: ROC Analysis
On the bottom of this page, you will find the topic for discussion and the name of the contributor.
Please add comments and then click on the "Add comment" button.
%COMMENT{type="belowthreadmode"}%
MaryBanach - 16 Nov 2011 - 14:49
I would like to suggest other areas and contributors to CTSpedia that may help inform this discussion. Our industry colleagues spend a great deal of time on ROC analyses for their submissions. We might want to invite Mac Gordon from Johnson & Johnson, who heads the Labs and Liver Safety Graphics (
ListingsLabsLiverVetted), and Rich Anziano from Pfizer, who heads the ECG/Vitals Safety Graphics (
ListingsECGVetted) to join us in our discussions. Also. I would like you to note the work that was done by Erin Esp, Laurel Beckett\x92s student at UC Davis, on a Clinical Research Case Study: Comparing Classification/Diagnostic Models (
DiagnosticsComparison).
3. Need to consider stages of testing
Pepe has a nice framework for this (
http://jnci.oxfordjournals.org/content/93/14/1054.full.pdf+html) (
http://jnci.oxfordjournals.org/content/93/14/1054.full.pdf+html>;;;;). ROC analysis does have a place in this multi-phase paradigm. Both the statisticians and clinicians have to start somewhere with the development and analysis. Our goal would be to comprehensively evaluate the marker and attenuate optimism early in the development. ROC and AUROC would be part of the report but not the entire report.
Rickey
That's where you are making a big a a leap Rickey, IMHO. It didn't follow that (1) tradeoffs were needed at publication time or (2) if tradeoffs were needed they should be derived from the patient's friends' and neighbors' characteristics, which is what ROCs do. The statistician's job is to make accurate risk or life expectancy predictions. Those predictions are self-contained for the purpose you are putting this to. Not only that but their error rates are self-contained. A predicted risk of 0.18, which if translated to a decision not to treat, means that you are wrong with probability 0.18. If you classify "treat" vs. "not treat" the true error probability is hidden. For example if a statistician playing the role of a decision maker came up with a rule to treat if the probability is greater than 0.25, the underlying error probability is hidden and may be as low as zero (if the probability of disease is 1.0) or as high as 0.25.
Best regards, Frank
2. Its utility depends on the field and purpose
In settings where a decision is to be made and acted upon immediately, there is much more need to reach a binary decision. AUC alone doesn’t help, but the ROC curve (or more precisely the data x,y pairs going into the graph) do help show the counter balancing of Sens, Spec, LR(T+), LR(T-) and the (diagnostic) odds ratio. I prefer these latter items tabulated as I think it is clearer for investigators. Nonetheless, the interpretation is in the figure. Predicting a future event is a different story—discrimination and calibration are critical.
Nicely put. AUROC is a good measure not because it is the area under an ROC curve but because it is the concordance probability which is an easy to interpret pure measure of predictive discrimination.
I have found this discussion to be very interesting and wanted to share a few thoughts. 1. AUROC is a useful summary metric
As with any “single number summary”, there are limitations, but in general, the AUROC is a good, general purpose summary of the discrimination of a continuous marker. Since it is directly related to the Mann-Whitney test and the concordance index, there are interpretations of the statistic beyond the averaged sensitivity interpretation. In my mind, it is a “table 1” sample summary akin to the sample mean. It helps sets the stage for more involved analyses down the road such as the covariate adjusted risk that Frank mentions.
These are excellent points - let me add one that shifts the focus from the physicians to the statisticians.
In some cases, we as statisticians may also contribute to the trend for dichotomization, when we restrict our presentation of statistical methods to those that can be applied to
- either "continuous" data (actually: having a linear relationship with the latent factor of interest -
ANOVA, linear regression, ...) - or binary data/outcomes (Mantel Haenszel, logistic regression).
I often hear that physicians are dichotomizing because they consider this a requirement for statistical methods to be applicable. We could help a lot if we would avoid oversimplifications (like "continuous" vs "categorical") in our teaching.
For instance, we could classify variables by their
- scale level (nominal, ordinal, interval, absolute) and - tie quality (exact: due to the nature of the phenomenon, inexact: due to discretization, including the choice of a discrete measurement for a continuous phenomenon) - granularity (2 vs more outcomes)
Then we could present methods as being applicable to different ranges of such variables. For instance,
- the t-test would require at least interval scaled or binary data, rather than "continuous" data, while - the u-test would be applicable to all scale levels above nominal, with the "correction for ties" appropriate for exact ties.
It may at first seem more burdensome to get concepts right from the beginning, but the long term benefit would be that our collaborators would not perceive statistics as forcing them to wear blinders (discretize their data) for the sake of being able to apply statistical methods.
Knut Sent via
BlackBerry by AT&T
That's a much better way of saying it. Disease severity or impact is really the issue, and should be emphasized over binary classification. We wouldn't have nearly the mess we have with prostate cancer diagnosis and decision making had we done that. A really good editorial is referenced below.
Frank
author = {Vickers, Andrew J. and Basch, Ethan and Kattan, Michael W.}
- title = {Against diagnosis}
- journal = Ann Int Med
- year = 2008
- volume = 149
- pages = {200-203}
- annote = {The act of diagnosis requires that patients be placed in a binary category of either having or not having a certain disease. Accordingly, the diseases of particular concern for industrialized countries---such as type 2 diabetes, obesity, or depression---require that a somewhat arbitrary cut-point be chosen on a continuous scale of measurement (for example, a fasting glucose level $>6.9$ mmol/L [$>125$ mg/dL] for type 2 diabetes). These cut-points do not ade- quately reflect disease biology, may inappropriately treat patients on either side of the cut-point as 2 homogenous risk groups, fail to incorporate other risk factors, and are invariable to patient preference.'' }
Link to the publication:
Great point. A lot of what we do assumes we know all of the influential factors. Unfortunately, I am not sure there is such a thing as a perect measurement and so I believe we have to use the evidence in the best way available until we have the full knowledge to interpret with 100% accuracy. I like the likehood ratio approach since it approximates how the test result might reasonably influence a physician's decision without making that decision in an abitrary manner, and it does not lose the continuous nature of the underlying variable.
I am not sure I fully agree that the diagnosis is rarely all or nothing. The truth is that the patient either has disease or does not. The question in my mind is disease severity, and whether or not there is a need to treat. This is not a question that ROC was designed to answer since severity of disease is not typically considered.
Chris
Frank,
your last point is so important. This is a sentiment I share frequently in journal clubs with my clinical colleagues - it worries me that physicians often renege on their responsibilities and rely on a binary cut point without understanding the implications. They really don't want me making decisions on their patients and only once I point out that's what they are allowing, it challenges them to think more deeply about the dichotomization.
One additional complexity to this discussion on utilities is that in some fields, shared decision making is really not possible. For example, in the severely injured patient, or the stroke patient. In these instances, the physician is hit with a sequence of problems: there is no time to gather additional test data and there is no possibility of having a conversation with the patient or often the family. Often the only data that becomes available is response to treatment, or lack thereof. The continually raging debate over tPA for stroke is a great example. On a group basis, there is no doubt of an overall survival benefit with improved functional outcome. On an individual basis, there is a real risk of intracerebral hemorrhage (6% if I recall correctly). This is a 'kill-or-cure' scenario in which the patient is rarely able to facilitate the discussion.
Chris
Thanks for the note Peter.
In my opinion one of the most misunderstood aspects of medical decision making is when exactly dichotomization needs to take place. A few observations:
- dichotomizing a predictor is two steps too early. It is easy to show that if a continuous predictor is dichotomized, its cutpoint will have to be a function of all of the other predictors in order to lead to a rational decision.
- dichotomizing a predicted risk in a statistical analysis or a manuscript, after effectively using all continuous predictors, is still one step too early if utilities are ignored
- the only necessary dichotomization is at the point at which the physician discusses all available information with the patient. This dichotomization is the treatment decision or decision to acquire more data. The optimum decision is a function of the probability of outcome and the utilities for taking all the possible actions.
Many statisticians make a leap of logic that dichotomizations should be done in a manuscript, i.e., that the medical decision needs to be made by the statistician using the statistician's (and not the patient's) utilities.
Best regards, Frank
This might be a good topic to make into a CTSpedia discussion thread, although discussion seems to be less robust there than by e-mail. (We probably need more people who are on this e-mail list to also be on the alert list for the discussion forum in CTSpedia.)
Also, I think it would be good to have one or more point-counterpoint type articles on CTSpedia that explore and elucidate controversial topics, so anyone please let me know (directly at
peter@biostat.ucsf.edu) if you might be interested in contributing to such an article on this topic.
To add a few more hornets about ROC analysis:
1. Dichotomization is necessary when a dichotomous decision must be made. In such cases, however, it seems irrational not to consider the stakes involved, i.e., the expected consequences of the available actions. This gap is addressed by the decision curve approach developed by Vickers. In addition to the reference that Frank already sent, a potentially useful website is
http://www.mskcc.org/mskcc/html/87831.cfm.
2. The area under an ROC curve is more abstract and difficult to interpret in terms of clinical importance than any one specific dichotomization. The appeal of avoiding reliance on any one specific cutpoint therefore has drawbacks as well as advantages.
3. Specifically, the abstract nature of AUROC worsens inherent problems in the power-based sample size approach: the arbitrary nature of the assumed goals and the sensitivity of the calculations to which goals are chosen (especially the effect size)
--Peter Bacchetti
Thanks for your note Chris. On the point about the likelihood ratio, doesn't that logic depend on the absence of other covariates, in addition to the usual assumption (rarely satisfied) that the diagnosis is all-or-nothing?
Frank
I agree that the usual approaches to ROC analyses are extremely limited, and ultimately lead to frequent misinterpretation of the data and poor decision making. However, there is one aspect that I find useful: the slope of the curve at any point is equivalent to the likelihood ratio (
http://www.ncbi.nlm.nih.gov/pubmed/9850136). This can really help to contextual the likely utility of a diagnostic test in practice.
Commonly used summary measures of ROC (e.g. the AUC, the point where sensitivity=specificity, or the point where the slope=1) I think are relatively useless. However, given the above relationship, a plot of the individual points with reporting of the threshold at those points can provide some useful information.
Chris
The main controversy is that ROC curves are divorced from and contradictory to optimum Bayes decisions and even optimum non-Bayes decisions in many cases. The frequency of use of ROC analysis does not make it right IMHO. Optimum decisions come from estimating risks, showing that the model is well calibrated, then incorporating patient-specific cost/loss/utility functions. ROC analysis just changes the subject. It tries to pretend that group decision making is useful for individual decision making.
Some good references are below.
Frank
author = {Vickers, Andrew J.}
- title = {Decision analysis for the evaluation of diagnostic tests, prediction models, and molecular markers}
- journal = The Am Statistician,
- year = 2008,
- volume = 62,
- number = 4,
- pages = {314-320},
- annote = {decision support techniques;outcome assessment;prognosis;decision theory;limitations of accuracy metrics;incorporating clinical consequences;nice example of calculation of expected outcome;drawbacks of conventional decision analysis, especially because of the difficulty of eliciting the expected harm of a missed diagnosis;use of a threshold on the probability of disease for taking some action;decision curve;has other good references to decision analysis}
- Link to Publication
author = {Fan, Juanjuan and Levine, Richard A.},
- title = {To amnio or not to amnio: {That} is the decision for {Bayes}},
- journal = {Chance},
- year = 2007,
- volume = 20,
- number = 3,
- pages = {26-32},
- annote = {decision theory;utility theory;Bayes decision tutorial;diagnosis}
author = {Bordley, Robert},
- title = {Statistical decisionmaking without math},
- journal = {Chance},
- year = 2007,
- volume = 20,
- number = 3,
- pages = {39-44},
- annote = {a graphical presentation of statistical decision theory;decision theory;graphics}
author = {Briggs, William M. and Zaretzki, Russell},
- title = {The skill plot: {A} graphical technique for evaluating continuous diagnostic tests (with discussion)},
- journal = Biometrics,
- year = 2008,
- volume = 64,
- pages = {250-261},
- annote = {ROC curve;sensitivity;skill plot;skill score;specificity;diagnostic accuracy;diagnosis;statistics such as the AUC are not especially relevant to someone who must make a decision about a particular $x_{c}$. \ldots ROC curves lack or obscure several quantities that are necessary for evaluating the operational effectiveness of diagnostic tests. \ldots ROC curves were first used to check how radio \emph{receivers} (like radar receivers) operated over a range of frequencies. \ldots This is not how most ROC curves are used now, particularly in medicine. The receiver of a diagnostic measurement \ldots wants to make a decision based on some $x_{c}$, and is not especially interested in how well he would have done had he used some different cutoff.''; in the discussion David Hand states when integrating to yield the overall AUC measure, it is necessary to decide what weight to give each value in the integration. The AUC implicitly does this using a weighting derived empirically from the data. This is nonsensical. The relative importance of misclassifying a case as a noncase, compared to the reverse, cannot come from the data itself. It must come externally, from considerations of the severity one attaches to the different kinds of misclassifications.''; see Lin, Kvam, Lu Stat in Med 28:798-813;2009}
author = {Hand, David J.},
- title = {Evaluating diagnostic tests: {The} area under the {ROC} curve and the balance of errors},
- journal = Stat in Med,
- year = 2010,
- volume = 29,
- pages = {1502-1510},
- annote = {diagnosis;diagnostic accuracy;fundamental problems with ROC area due to failure to balance difference kinds of misdiagnoses effectively;proposal to use H index discussed in Hand DJ:Machine Learning 77:103-123;2009}
- Link to Publication
Rao Marepelli - 6 Nov 2011
Let us stir up the hornet's nest. I have an armload of papers and a couple of books on ROC curves all glorifying the edifice of ROC analysis. I really want to know where the controversy lies. MB Rao
Just to stir up a hornets nest ROC analysis is quite controversial and often is associated with a loss of power. ROCs also lead people to use cutoffs which we know is a dangerous statistical practice.
Frank
Rao Marepelli - 6 Nov 2011
Chris, I am looking forward to the face-to-face meeting to be organized next year. I have been working on a number of methodological and practical issues stemming from my CCTST activities. I have a number of things to report. A sampler:
1. ROC analysis and sample size calculations
2. Cronbach Alpha and Bootstrap
3. HCUP data and what it can give us
May be one of these could fit into one of the didactic sessions!
MB
This topic: CTSpedia
> WebHome >
DiscussionForum > DiscussionBERD003
Topic revision:
09 May 2013, MaryBanach
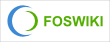
Copyright © by the contributing authors. All material on this collaboration platform is the property of the contributing authors.
Ideas, requests, problems regarding Foswiki?
Send feedback